Building data-driven organizations, Part 3: How to operationalize better decision-making
A simple process for creating lasting change
This post is part 3 of a 3-part series on building data-driven organizations. Part 1 is here and part 2 is here.
Let’s recap what we’ve covered so far. Part 1 explained why being data-driven means making decisions differently based on data. Part 2 introduced the abstraction of companies as factories that produce decisions, just like real factories produce widgets and other goods. Data-driven organizations are well-run factories that efficiently produce high-quality decisions.
How do we run our factories more effectively?
We started to answer this question by applying the 5 Why’s to real examples of decisions. We identified the most common reasons companies fail to make data-driven decisions. It’s similar to finding the parts of the assembly line that cause the most production problems (and potentially drive the most improvement).
But real change occurs when knowledge translates to everyday actions. The best factories have systems that continuously monitor their production quality. Analytics leaders should apply a similar operational mindset to decisions made in their organizations.
In this final piece, I introduce a simple process you can embed into your existing workflows to do exactly that: operationalize better decision-making.
1. Develop a scorecard
Like most things in business and life, you don’t improve what you don’t measure. Measurement gives us the ability to track progress, set goals, and hold people accountable to an objective standard. Decision-making works the same way: making an organization more data-driven starts with measuring it.
But unlike a metric like conversion or active users, “data-drivenness” isn’t a quantity we can easily throw on a dashboard and set goals against. In theory, we can keep track of how every decision gets made, but it’s impractical because most decisions cannot be easily recorded.
Instead, we use a scorecard that evaluates data-drivenness across key attributes that we borrow from the most common categories of decision-making failures:
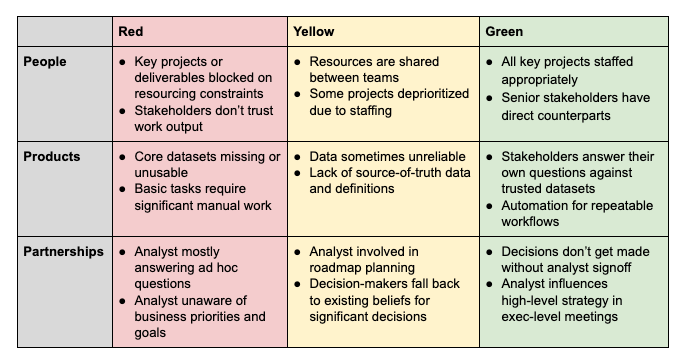
Like in a career pathway, the boxes show progression across each attribute and the individual bullets represent example behaviors. We make more data-driven decisions as we improve along each attribute.
This scorecard is a template and you should treat it as such by adapting it to your own needs. As you encounter new decision-making challenges over time, refine the criteria, scale, and even attributes. In the end, it’s important that the scorecard reflects your own view of what success means.
2. Build a review process
Areas of improvement (indicated by red boxes on the scorecards) are not usually surprises. But unfortunately, teams make little meaningful progress because they fail to prioritize the problems. 6 months goes by and the same problems resurface, potentially when things have gotten worse.
This happens because as analysts, we’re used to spending most of our time and energy tending to immediate business needs. The problems in the scorecard require the same amount of attention, but often fall into the “important but nonurgent” column of things we never get to work on.
One key to improving data drivenness is to act on the scorecard regularly by establishing a process for evaluating, discussing, and taking action. An organizational habit, so to speak, that creates frequent, consistent space to review the scorecard and hold people accountable.
Review scorecards
Make a copy of the scorecard and review it with each direct report at least once a month. Use the time to evaluate the scorecard, discuss challenges, and identify solutions. For example, if the score for “products” is red because some core reporting is broken, find the underlying cause and agree on next steps. The answer might be that a particular dataset need updating, which would lead to concrete next steps.
Scorecard reviews are also opportunities to calibrate expectations with your team. A team member may think he is green in a particular category, but based on your observations he is only at a yellow.
Generate a heatmap
After reviewing each team member’s scorecard, aggregate the individual scorecards into a heatmap. This brings problems (and bright spots) into sharper focus by visually distinguishing between isolated issues and systemic ones affecting multiple people.
For example, if one analyst is unable to convince her product manager and engineers to prioritize fixing event tracking bugs, there might be any number of reasons why. But if many analysts have the same problem with different teams, there may be a broader cultural or technical problem to take up with product and engineering leadership.
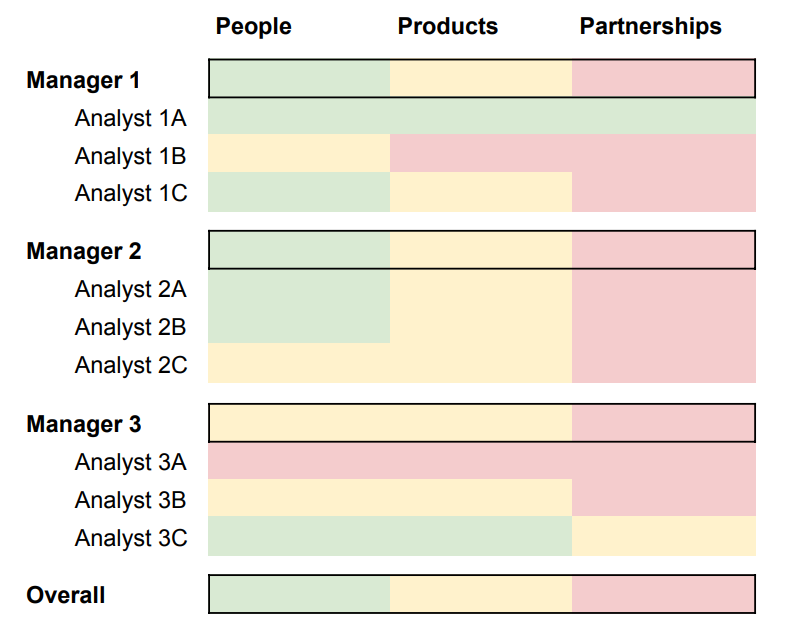
Cascade up the chain
In larger data teams, managers of managers can ask direct reports to present their scorecards at staff meetings. An open forum allows managers to share their challenges, successes, and learnings with peers who encounter similar situations.
Scorecards also cascade up the hierarchy and generate an overall heatmap for the entire team. A teamwide heatmap accomplishes several things. First, it helps line managers benchmark themselves against their peers. Secondly, it clarifies responsibility by showing where the problems lie within the team. Lastly, it provides a tool for leaders to identify and prioritize the important issues as they bubble up across the organization.
3. Communicate the vision
Committing to a regular review process creates extra work for your team. The key to success is ensuring your team is bought in every step of the way. Help them understand why it’s important and describe your vision. The ideals of building a more data-driven organization may seem obvious to you, but don’t take it for granted that everyone will immediately get it.
Create safety
New scoring systems can potentially breed resentment and defensiveness. Recognize and pre-empt this concern, which undermines the team’s ability to have open, productive conversations.
Unlike a performance review, the sole purpose of the scorecard and management process is to help teams become more data-driven. It’s not a competition and there are no winners. Teams that create space to tackle these problems together benefit from collaboration and see faster progress. Remind your teams of these points frequently and create the psychological safety they need.
Define success
If you want to build a data-driven organization, you need to show your team what it looks like. In a way, your definition of success is reflected in the “green” behaviors in your scorecard. But words on a page only goes so far: elaborate on the bullet points and paint a vision of what the world should feel like.
For example, there are different interpretations of what “automation for repeatable workflows” means. What kind of automation? Which workflows? Clarify the vision: we’re building toward a world in which no analyst will ever have to setup or analyze an experiment manually again.
Or the bullet “analyst influences high-level strategy in exec-level meetings”. Use an example of a strategic decision or meeting in your company to show your team what influence means.
In my experience, a leader’s vision is often grander than what the team initially believes. Tell people about the promised land, and it’ll become much easier to lead them there.
Embrace the journey
If you follow these steps, you’ll see measurable progress. You’ll see scorecards moving from red to yellow to green. You’ll see improvements in team productivity and stronger relationships with business partners.
But you’ll also move in the wrong direction sometimes. All of a sudden you need to hire people with specialized skill sets. The data infrastructure you used previously stopped scaling. A significant reorg altered your relationship with a partner team for the worse.
Setbacks are normal because what companies need to make data-driven decisions are constantly evolving. Help your team understand that building a data-driven organization is not a race to a finish line, but a continuous pursuit of excellence. There are ups and downs along the way. Remember to stay motivated and embrace the journey.
Wrapping up
Data-driven organizations are not built overnight. They are forged over years of slowly chipping away at problems and cementing data into the decision-making process. They are not defined by paper achievements or technology choices, but by their steady refinement of decision-making as a craft. From time to time they produce faulty decisions, but on the whole they are consistent and resilient.
Every company has the potential to become a data-driven organization. Over the last 3 posts, I shared a few key mental models and tools that can help you get there. We discussed what it means to be data-driven, how to diagnose decision-making problems, and how to apply these frameworks in your everyday work. These tools have been influential in my own journey. I hope they can also be useful in yours as well.
Good luck!
Thank you, very helpful!